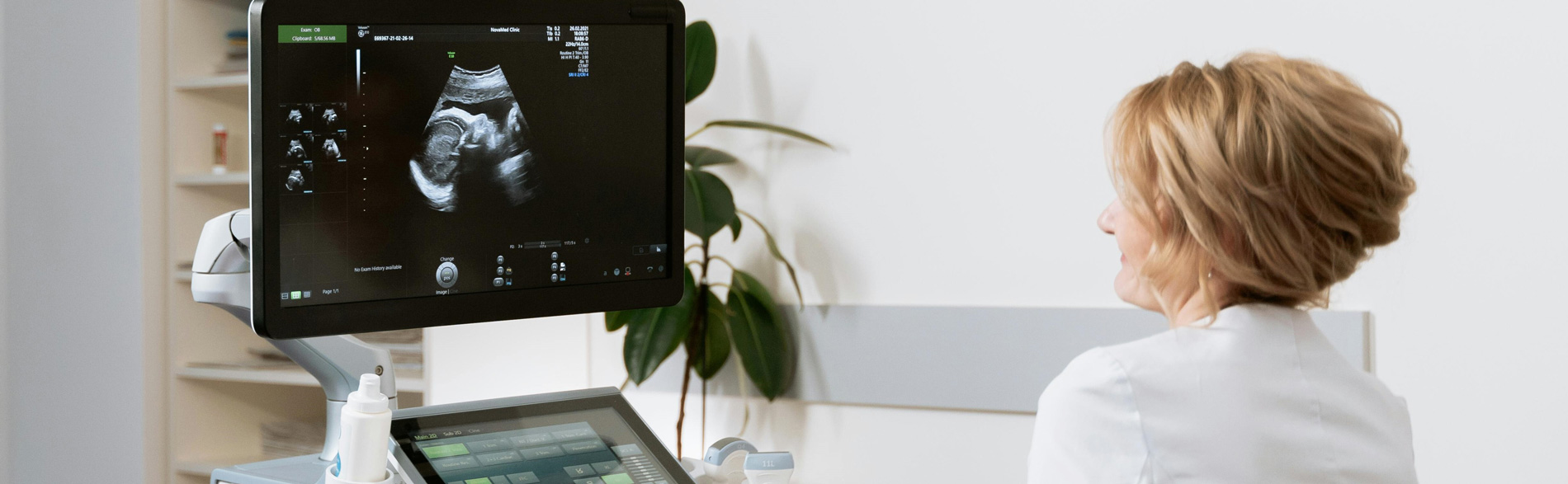
AI in healthcare
Authors: Debjyoti Karmakar and Fiona Brownfoot
A multidisciplinary perspective from obstetrician-gynaecologist clinician-researchers
Artificial intelligence (AI) in healthcare has progressed from the Greeks' mechanistic automatons to today's sophisticated capabilities. This transformation is notable in Obstetrics and Gynaecology, where we have evolved from simpler, non-generative machine-learning models to advanced deep-learning frameworks. These newer, generative AI models enable a nuanced understanding of personalised care.
Despite slower adoption in women's health compared with fields such as oncology or chronic disease management—primarily due to disparities in research funding—AI holds significant potential for addressing complex clinical challenges. Established techniques such as linear and logistic regression provide a robust statistical foundation for understanding women's and foetal health outcomes like other clinical areas. However, there is growing interest in more sophisticated machine learning tools, such as nearest neighbour and support vector machines (SVMs), which improve our ability to identify successful treatment pathways and delineate disease patterns efficiently. Decision trees, random forests and neural networks have significantly expanded AI's typology, particularly enhancing predictive analytics in healthcare and thus have significant potential in perinatal medicine.
Scalability of AI in women's health
Our lab is uniquely positioned in Australasia – it pioneers the philosophy of leveraging big data and intelligent signal processing to develop AI-driven solutions for foetal monitoring, perinatal asphyxia detection and endometriosis diagnostics. Notably, despite advancements in diagnostic technologies for these conditions over the last decade, there has not been a corresponding improvement in health outcomes or reduction in the societal disease burden. AI can change this by better handling multidimensional and multimodal data. For the challenge of perinatal asphyxia, we are using deep learning models trained on a repository of half a million cardiotocography time-series datasets. We are also developing a novel, non-invasive foetal monitoring sensor that uses AI for enhanced signal processing and filtering. Traditional statistical and epidemiological models are inadequate in analysing such extensive, complex data sets, highlighting the necessity of advanced AI algorithms for effective interpretation.
The conundrum around generative AI
The emergence of generative AI, including large language models (LLMs), marks a significant evolution in the field, capable of creating new content such as images, music, text, and complex simulations. Unlike discriminative models that classify data and make predictions, generative models can produce new examples within a dataset's distribution. This capability is promising in synthesising medical data and generating insights that could lead to better diagnostics, personalised medicine and innovative treatment strategies. It could theoretically predict interactions between chemical compounds, generate synthetic medical images for training radiologists, women’s health sonologists and specialists in women’s imaging without privacy concerns, and improve the construction of 3D models from 2D images. Nevertheless, this innovation comes with challenges, including concerns about patient privacy, data security and the integrity of synthesised data, which are crucial to prevent misdiagnoses and ensure treatment effectiveness. Regulatory bodies also face difficulties approving AI-generated diagnostics and treatments, requiring clear evidence of their safety and efficacy.
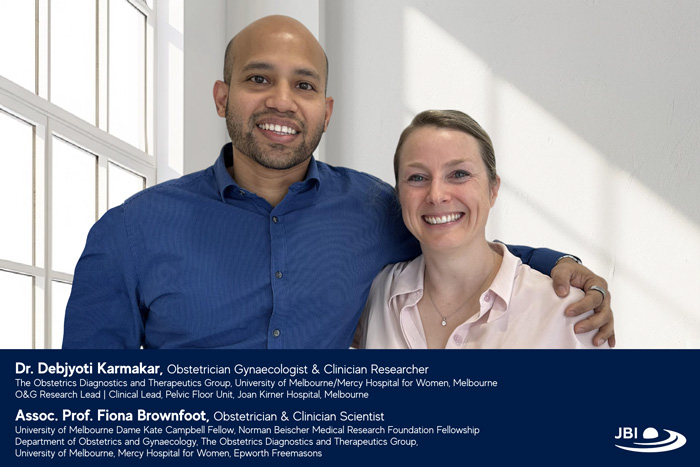
As we advance the application of AI in obstetrics and gynaecology, we must uphold rigorous ethical standards to ensure our technological progress aligns with our core human values. The future of AI in healthcare depends not just on sophisticated algorithms and data processing capabilities, but also on our steadfast commitment to align these innovations with trust, empathy and equity in patient care.
Authors
Dr Debjyoti Karmakar
Obstetrician Gynaecologist & Clinician Researcher
(NHMRC PhD Scholarship)
The Obstetrics Diagnostics and Therapeutics Group
University of Melbourne/Mercy Hospital for Women
O&G Research Lead | Clinical Lead, Pelvic Floor Unit, Joan Kirner Hospital
Melbourne, Australia
Assoc Prof Fiona Brownfoot
Obstetrician & Clinician Scientist
University of Melbourne Dame Kate Campbell Fellow
Norman Beischer Medical Research Foundation Fellowship
Department of Obstetrics and Gynaecology
The Obstetrics Diagnostics and Therapeutics Group
University of Melbourne, Mercy Hospital for Women
Melbourne, Australia